User Guide
Descriptive statistics
Albert Einstein once said, "God does not play dice", when talking about quantum mechanics, calling into question one of the bases of modern physics. That being said, the experiments carried out in the 20th century proved him to be wrong as it has been admitted that the whole of observable phenomena in the world are governed by distribution properties that are all subject to the Heisenberg uncertainty principle. The world is random…
Understanding random phenomena is fundamental to understanding how the world works. However, we can mathematically represent a central statistical data analysis theorem: the central limit theorem.
Any system resulting from the sum of numerous factors, independent of each other and of the same magnitude, generates a distribution law that leans towards normal distribution.
This theorem shows how normal distribution is important in the variability analysis of observable phenomena. To illustrate this, let us roll one die 1000 consecutive times and observe the results.
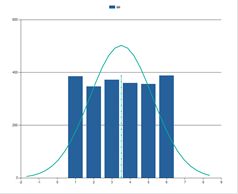
The distribution follows a uniform law, i.e. there are as many chances that the dies will fall on 1, 2, 3, 4, 5, or 6. The law of distribution does not resemble normal distribution.
Now we shall roll 10 dice 1000 consecutive times and observe the results distribution from the sum of these 10 dice:
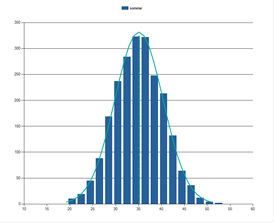
While each die follows a uniform distribution, the distribution of the sum of the 10 dice follows a bell curve. This distribution closely follows normal distribution.
Actually, if we follow what is given under the central limit theorem:
- We get a system
- Resulting from the sum of numerous factors (the sum of 10 dice)
- Independent from each other (the result of one die has no influence on the result of the other dice)
- The order of magnitude of each die is equivalent
The distribution generated by this system leans towards a normal distribution. On a whole this is intuitive. Whenever 10 dice are rolled there is no one combination that will yield a result of 10 (all of the dice come up 1) while there are thousands of combinations that will yield a result of 35. Consequently, those results close to 35 have a higher probability of yielding extreme results such as 10 or 60. The resulting distribution is therefore close to a normal distribution.
This is what we generally find, systems that we often observe have this type of distribution as they fit the hypothesis of the central limit theorem. Let us use the example of machining a part:
- The system in question produces a rating.
- The deviation of the rating based on the target produces the sum on numerous factors (vibration, hardness, tool position error, etc...)
- The factors are independent from each other (machine vibrations have no effect on material hardness)
- The order of magnitude of these deviations is equal
The parts distribution therefore tends towards a normal distribution, which is what we observe when measuring a series of parts.
Ellistat supplies the main descriptive analysis tools necessary to analyse capability and to describe the statistical behaviour of a variable so that you can analyze your measurements.
- Spreadsheet
- Summary
- Details
- Off tolerance proportions
- Continuous distribution
- Discontinuous distribution
- Attributes chart