User Guide
Parametric vs non-parametric tests
When carrying out population comparisons where a population is being compared with a theoretical value, there are two large families of tests: parametric and non-parametric tests
Parametric tests
Parametric tests function under the assumption that given data follows a known distribution law (generally normal distribution).
In order to calculate the alpha risk of a statistical test, all that needs to be done is calculate the sample's mean and standard deviation to access the sample's distribution law.
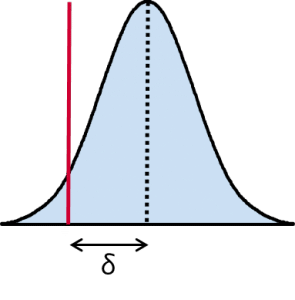
Because the distribution law is perfectly known, the alpha risk can be calculated based on the theoretical calculations of the Gaussian law.
These tests are generally very precise, but they require that the data efficiently follows the assumed distribution law. They are particularly sensitive to abnormal values and it is not recommended if abnormal values are detected.
Non-parametric
Non-parameteric tests do not hypothesise the type of distribution law for given data. They are solely based on the numerical properties of the samples. Here is an example of a non-parametric test:

We want to verify the median for a population that differs from the theoretical value. We measure 14 parts to obtain the following sample:
11 out of 14 times; the result is less than the theoretical median If the median for the population is equal to the theoretical value then 50% of the parts be above the median and 50% below the median. In order to make a claim about the significance of the difference between the median and the theoretical median, all that needs to be done is verify if the frequency 11 out of 14 times is significantly different than 50%.
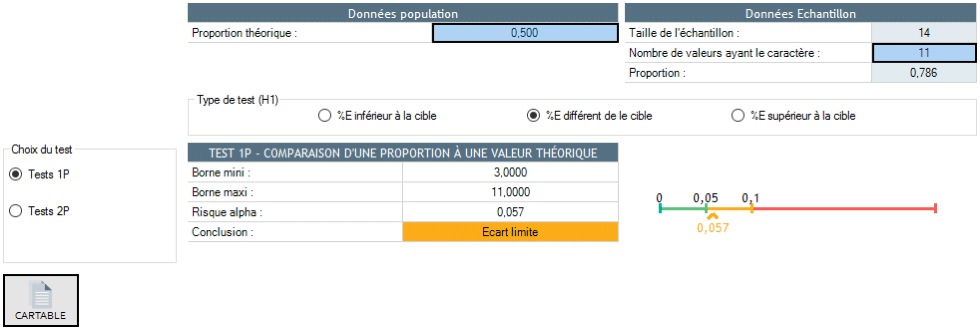
We note that this difference is borderline.
As in the preceding example, the non-parametric tests do not need an assumption of a particular distribution type in order to calculate the test's alpha risk. They are very elegant tests based on numerical properties. Moreover, they are more sensitive to abnormal values and are therefore recommended in this case.